Associated Sub-Fields:
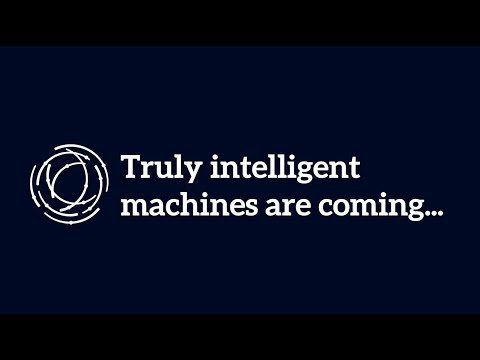
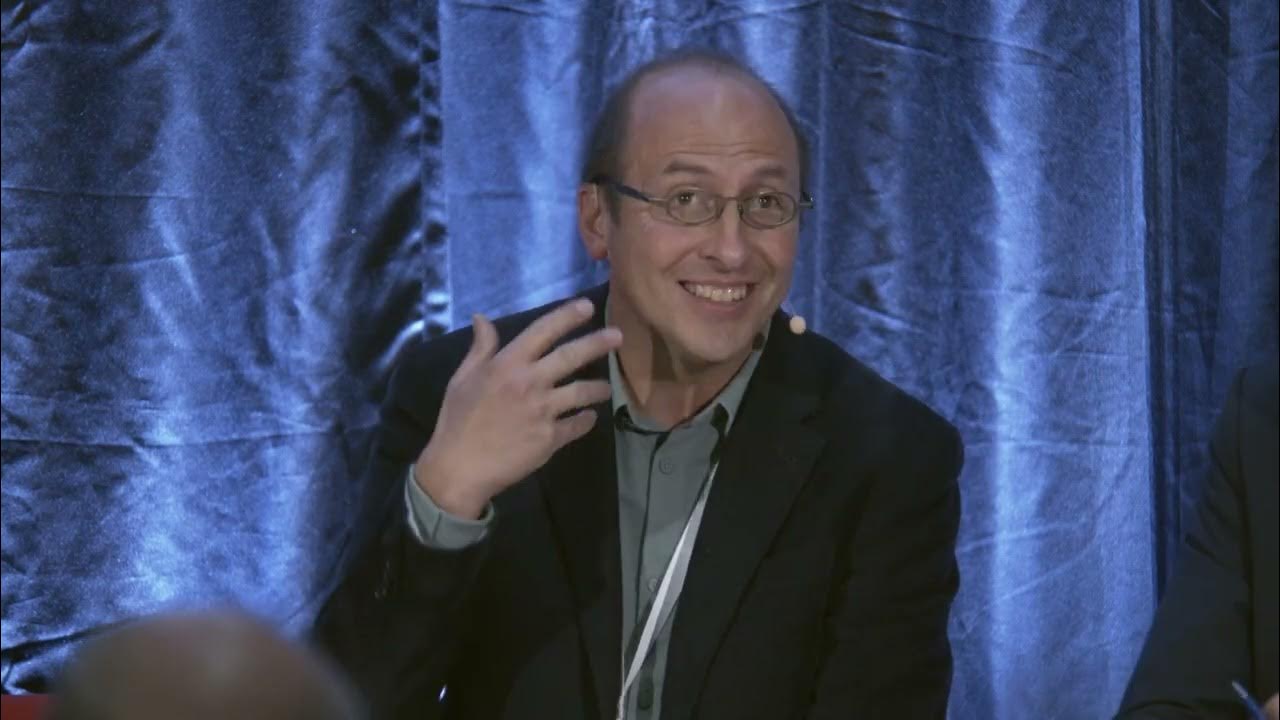
At the core of AI lies machine learning (ML), the methodologies allowing machines to learn and make predictions autonomously by discovering patterns in data. Deep learning is an ML methodology that relies on large models called neural networks, in particular transformers. When fed large amounts of data, neural networks learn to recognise statistical patterns, which can then be used to solve a variety of tasks in areas such as vision, language or game-playing.
Computer vision systems powered by deep learning identified objects better than humans in 2015.1 The following year, deep-learning systems beat a Go champion and started playing complex video games.2 Last year, AI predicted the structure of nearly every known protein and brought chatbots with powerful linguistic capabilities to the general public.34 Today, a wide range of AI tools including chatbots, image generators and coding assistants are commercially available and even integrated into search engines and office software.
This rapid progress is primarily due to the increasing availability of data and computing power. There is evidence that AI capabilities scale with model or dataset size leading many AI research labs and organisations to focus on building ever-larger systems.5,6 However, deep-learning-based AI remains prone to bias and often can’t generalise what it has learned to new situations, an ability known as out-of-distribution generalisation. The inner workings of neural nets are also opaque and they are inefficient learners, requiring vast amounts of data, processing power and energy. This has led to predictions that improved approaches will be required to achieve more adaptable, efficient and trustworthy AI that can be deployed broadly across society.7 That said, many in the field believe that continued scaling could also address these outstanding challenges to an extent that is acceptable in many applications, and that society needs to prepare for the disruptive effects of AI that can outperform humans in a wide range of tasks.
KEY TAKEAWAYS
Recent progress in AI research has already had enormous societal impact, and more is certain to follow. Advances known as Deeper machine learning offer significant improvements on current capabilities, leading to rapid improvements in language, coding, vision and robotic control, although bias and “hallucination” of untruths and weakness of reasoning remain a problem. Future AI systems are increasingly likely to use data from a variety of source types. Such Multimodal AI is already in limited use in autonomous vehicles, and will spread to a much wider set of applications. This is likely to accelerate its learning and to improve human-computer interaction, since humans are themselves multi-modal. Concerns over privacy, bandwidth and safety mean that research is exploring the use of Intelligent devices that can process data and run AI models on smaller platforms. The challenges here are spurring innovations in chip design, training and inference protocols. There are hints that scaling, resource use and trustworthiness issues associated with current AI may hinder its full development, necessitating new methodological development of Alternative AI.
Anticipatory Impact:
Three fundamental questions guide GESDA’s mission and drive its work: Who are we, as humans? How can we all live together? How can we ensure the well-being of humankind and the sustainable future of our planet? We asked researchers from the field to anticipate what impact future breakthroughs could have on each of these dimensions. This wheel summarises their opinions when considering each of these questions, with a higher score indicating high anticipated impact, and vice versa.
- Anticipated impact on who we are as humans
- Anticipated impact on how we will all live together
- Anticipated impact on the well-being of humankind and sustainable future of our planet
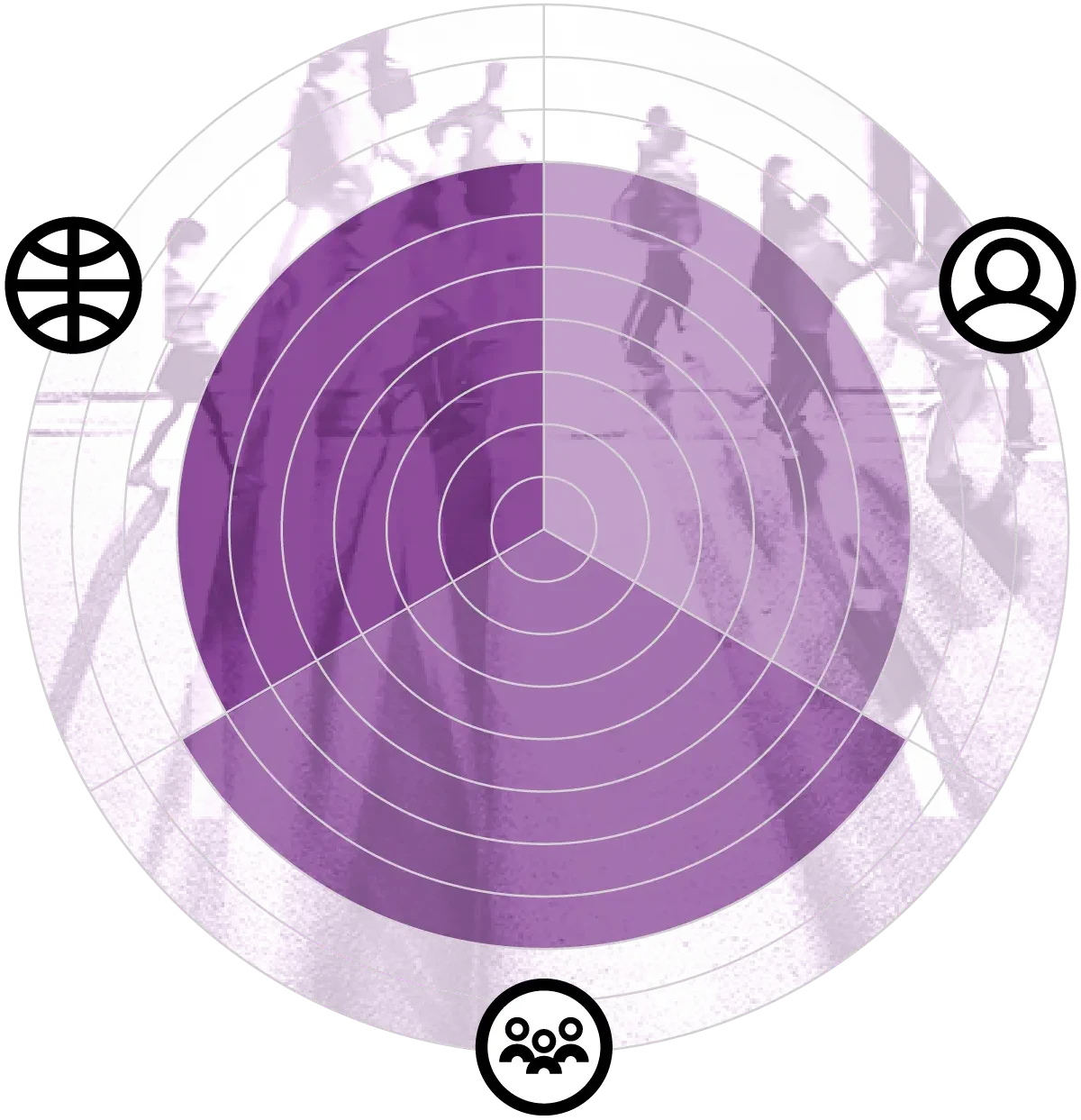