Future Horizons:
10-yearhorizon
Autonomous robot deployment expands
25-yearhorizon
Humanity experiences ambient intelligence in the environment
Hence, running models embedded on “intelligent devices” will be imperative for many of the most promising AI applications.28 However, smaller machines with limited power supplies are unable to support the computing and energy requirements of today’s leading models. This is spurring efforts to develop more efficient chips specialised for embedded applications, and optimisation techniques that can make models smaller, more energy-efficient and faster.293031 Analogue AI chips are showing promise as a way to dramatically reduce the power required to run leading models.32
Training these intelligent devices will also require innovations. Federated learning is a promising approach that distributes training over many smaller devices, reducing bandwidth requirements and improving privacy.33 Reinforcement learning, in which AI learns to perform a task by repeatedly performing actions to maximise a carefully chosen reward, will keep improving the training of robots and other automated machines. This is slow and costly, though, and collecting enough real-world training data is a challenge. New open robotics datasets can help researchers pool resources, but training models in simulations and then porting them over to devices may work better.34
Intelligent devices - Anticipation Scores
The Anticipation Potential of a research field is determined by the capacity for impactful action in the present, considering possible future transformative breakthroughs in a field over a 25-year outlook. A field with a high Anticipation Potential, therefore, combines the potential range of future transformative possibilities engendered by a research area with a wide field of opportunities for action in the present. We asked researchers in the field to anticipate:
- The uncertainty related to future science breakthroughs in the field
- The transformative effect anticipated breakthroughs may have on research and society
- The scope for action in the present in relation to anticipated breakthroughs.
This chart represents a summary of their responses to each of these elements, which when combined, provide the Anticipation Potential for the topic. See methodology for more information.
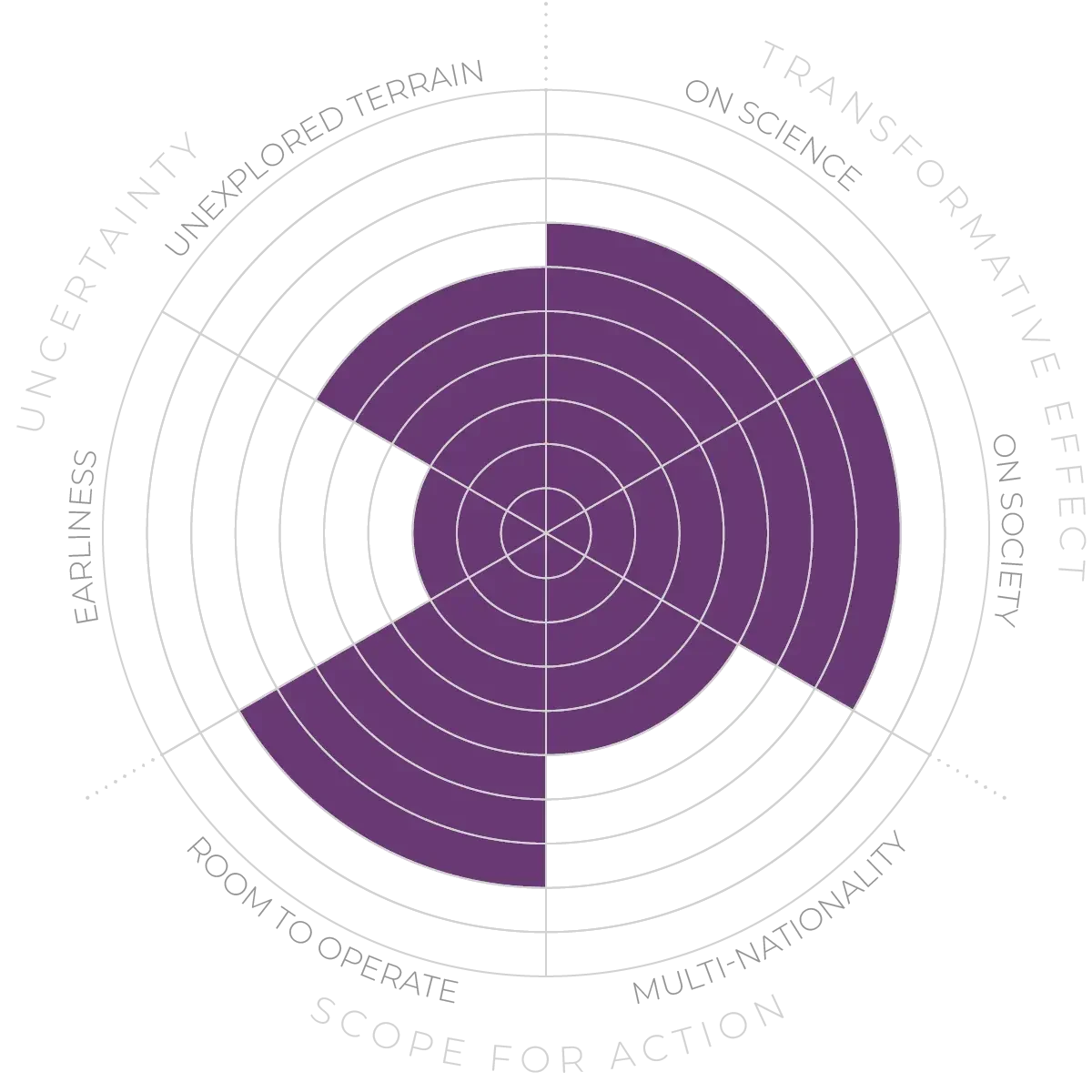