Future Horizons:
10-yearhorizon
Workforce disruption necessitates radical intervention from policymakers
25-yearhorizon
Deep learning’s influence is ubiquitous in daily human life
This has led to rapid improvements in AI language and coding capabilities and is also showing promise in domains like vision and robotic control.91011 So far, transformer performance scales reliably with model or dataset size, and the largest systems appear to demonstrate emergent capabilities they were not explicitly trained for, such as some creativity and limited reasoning capabilities in language.12 They have also been applied to scientific problems such as protein folding with considerable success.13
Nonetheless, there is evidence that the current “scale” approach is learning mainly by memorisation, exemplified by poor performance on more complex tasks such as mathematics and logic.14 Research has also suggested that seemingly emergent capabilities are simply the result of flawed testing.15 And because these models are statistical in nature, they readily learn biases from training data and can confidently “hallucinate” facts that are not true.16
The imperative to build ever-larger models also means cutting-edge AI research is increasingly accessible only to well-funded labs possessing significant compute power. There is also interest in creating smaller models,17 but access to data and hardware remains a key differentiator and is stoking geopolitical competition over access to AI chips.18
Deeper Machine Learning - Anticipation Scores
The Anticipation Potential of a research field is determined by the capacity for impactful action in the present, considering possible future transformative breakthroughs in a field over a 25-year outlook. A field with a high Anticipation Potential, therefore, combines the potential range of future transformative possibilities engendered by a research area with a wide field of opportunities for action in the present. We asked researchers in the field to anticipate:
- The uncertainty related to future science breakthroughs in the field
- The transformative effect anticipated breakthroughs may have on research and society
- The scope for action in the present in relation to anticipated breakthroughs.
This chart represents a summary of their responses to each of these elements, which when combined, provide the Anticipation Potential for the topic. See methodology for more information.
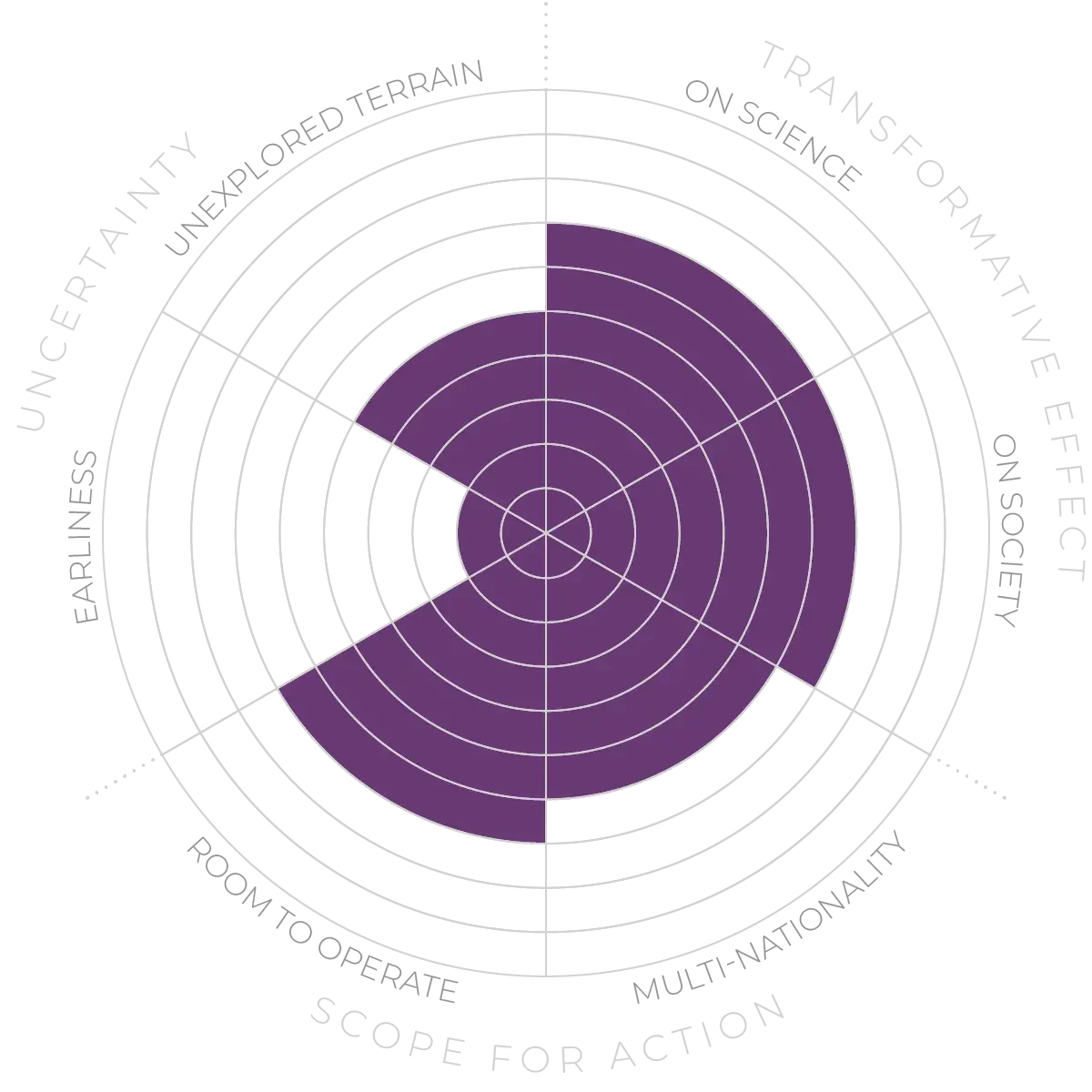