Future Horizons:
10-yearhorizon
Emerging scaling laws determine the future of deep learning
25-yearhorizon
Deep learning continues to dominate
Alternative approaches could solve some of these issues. Neurosymbolic AI, for instance, combines statistical learning with structured representations of prior knowledge, often inspired by cognitive science. It appears more capable of common-sense reasoning, and decisions are more readily interpretable by humans.36 Google DeepMind recently used the approach to solve complex geometry problems at the level of human experts.37 While deep learning focuses on finding correlations in data, Causal AI seeks to give machines a deeper understanding of cause and effect that could be crucial for general intelligence.38 And Bayesian AI approaches use the statistics of probability to help AI better understand the uncertainty of the real world while achieving continuous learning.39
So far, these approaches have not achieved the same success as deep learning. Many researchers also believe that similar capabilities will emerge spontaneously as deep-learning models become larger and more sophisticated. But our theoretical understanding of deep learning lags far behind the practice, making it difficult to spot potential roadblocks to progress. It is possible that some combination of these approaches may be necessary if we want to recreate the kind of general intelligence seen in humans.
Alternative AI - Anticipation Scores
The Anticipation Potential of a research field is determined by the capacity for impactful action in the present, considering possible future transformative breakthroughs in a field over a 25-year outlook. A field with a high Anticipation Potential, therefore, combines the potential range of future transformative possibilities engendered by a research area with a wide field of opportunities for action in the present. We asked researchers in the field to anticipate:
- The uncertainty related to future science breakthroughs in the field
- The transformative effect anticipated breakthroughs may have on research and society
- The scope for action in the present in relation to anticipated breakthroughs.
This chart represents a summary of their responses to each of these elements, which when combined, provide the Anticipation Potential for the topic. See methodology for more information.
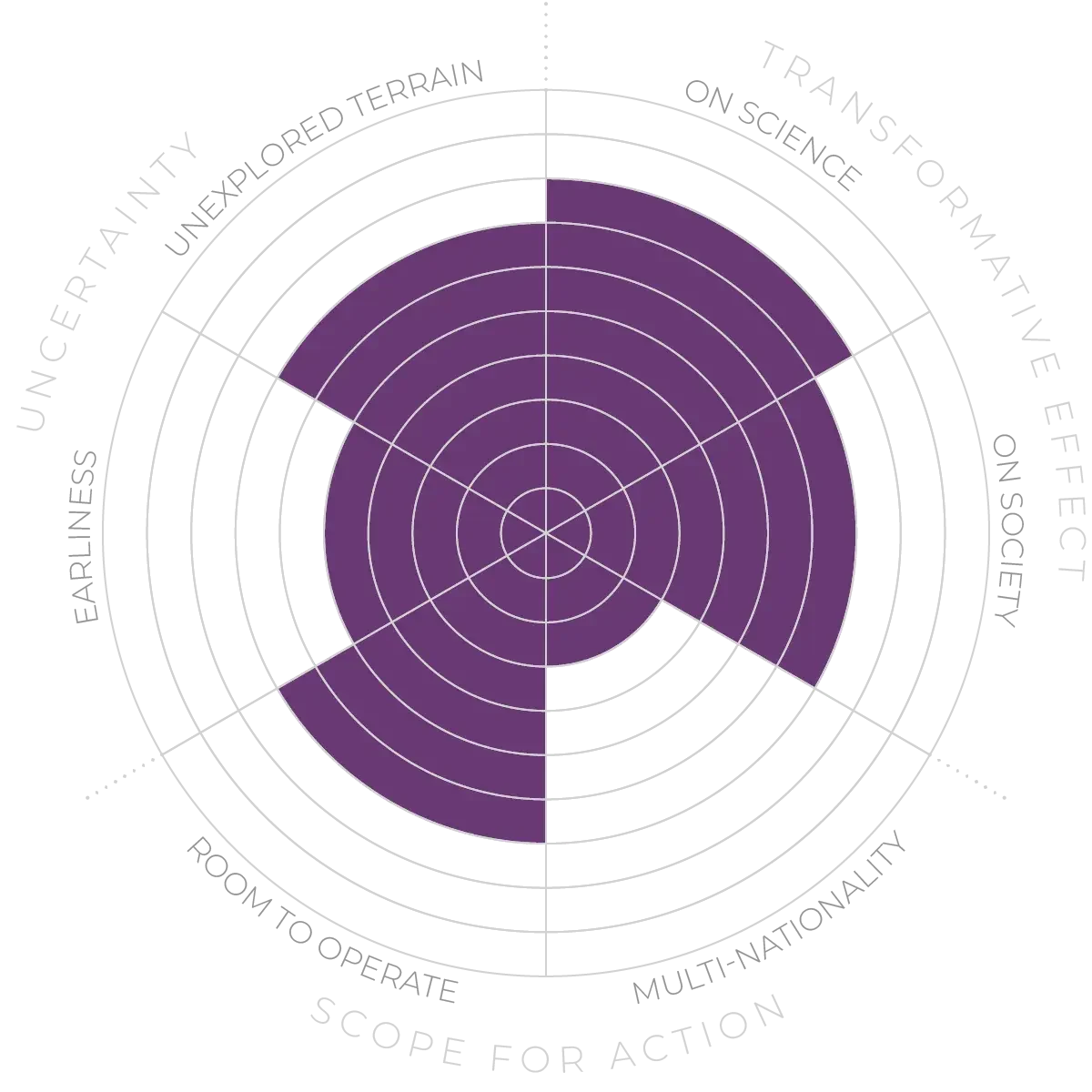