Future Horizons:
10-yearhorizon
A virtuous circle eases data woes
25-yearhorizon
The data crunch is over
Transformer-based AI presents both opportunities and fresh problems. Transfer learning — the ability to take a model trained on one kind of robot and deploy it on another — has been a long-standing challenge.31 Even small changes in environment or robot configuration can throw models off, meaning robots have to be individually trained. Transformers are able to train on data from multiple robots to create more general policies that work across varied embodiments and environments.32. However, training one of these models requires colossal amounts of data.
One potential workaround involves training models in simulations before porting them over to real-world robots.33 But many of the tasks planned for robots, such as handling soft and delicate objects, are incredibly hard to simulate.34 Designing virtual worlds is also complicated and expensive, requiring sustained effort from multidisciplinary teams.35
Pooling data-collection efforts will be crucial going forward, and there are promising efforts to create massive open robotics datasets.36 There will also be a growing focus on more data-efficient training approaches.37 Data shortages are likely to ease as more robots are deployed, though this will benefit the private companies that build them more than academic researchers.
Data - Anticipation Scores
The Anticipation Potential of a research field is determined by the capacity for impactful action in the present, considering possible future transformative breakthroughs in a field over a 25-year outlook. A field with a high Anticipation Potential, therefore, combines the potential range of future transformative possibilities engendered by a research area with a wide field of opportunities for action in the present. We asked researchers in the field to anticipate:
- The uncertainty related to future science breakthroughs in the field
- The transformative effect anticipated breakthroughs may have on research and society
- The scope for action in the present in relation to anticipated breakthroughs.
This chart represents a summary of their responses to each of these elements, which when combined, provide the Anticipation Potential for the topic. See methodology for more information.
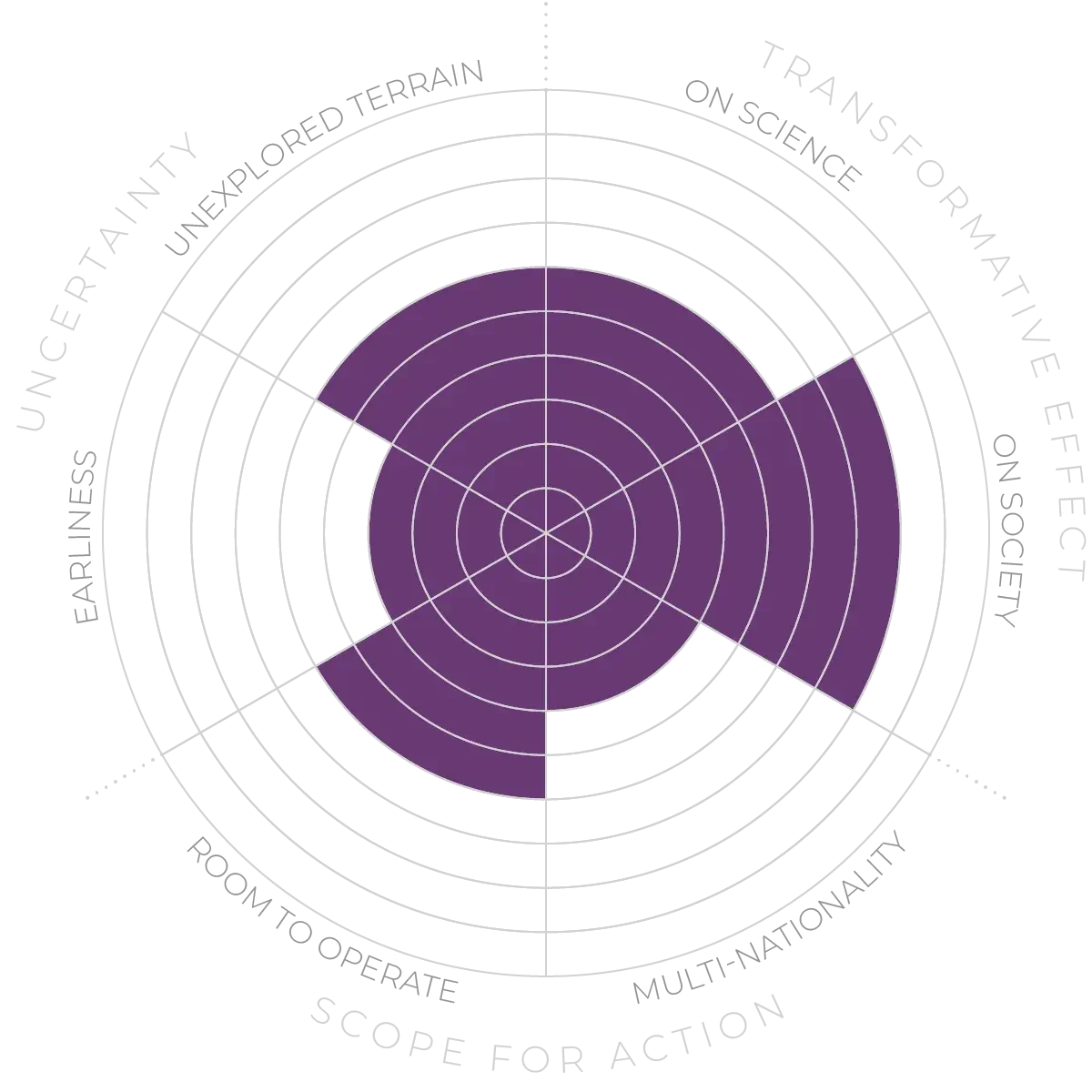