Future Horizons:
10-yearhorizon
Quantum chemistry comes of age
25-yearhorizon
Medical quantum-computing algorithms proliferate
Already of interest is the use of quantum computers to perform quantum simulation. Because they follow the same rules as physical entities on the atomic and subatomic scales, quantum computers can enable discovery and mimicry of the characteristics and dynamical processes of these materials, aiding pure science discovery as well as technological innovation. The most useful calculations of this type are intractable on classical computers. One example is calculating the quantum states of molecules involved in chemical energy storage, which will improve battery technology. Similarly, the details of various chemical reactions involved in the efficacy of pharmaceutical drugs are beyond the capabilities of classical computing, but may be achievable on quantum computers with relatively few (perhaps around 100) physical qubits. Other healthcare and life-science applications are also envisaged.10 Many quantum algorithms for quantum simulations use both quantum computers and classical ones in an integrated manner. This is because only part of a very complex molecular simulation benefits from quantum algorithms; the rest of it can be performed by conventional simulation methods, including machine-learning techniques. This framework is known as hybrid quantum computing and it is considered nowadays the only path to scalability.
There is also increasing interest in using quantum computers to assist machine-learning algorithms11 and financial modelling.12 Similarly, logistics applications, such as routing and scheduling, may benefit from the advantages offered by quantum search algorithms. As well as mathematically rigorous algorithm design, there is scope for heuristics, where quantum computing advantages can be achieved through trial-and-error approaches.
Quantum Algorithms design - Anticipation Scores
The Anticipation Potential of a research field is determined by the capacity for impactful action in the present, considering possible future transformative breakthroughs in a field over a 25-year outlook. A field with a high Anticipation Potential, therefore, combines the potential range of future transformative possibilities engendered by a research area with a wide field of opportunities for action in the present. We asked researchers in the field to anticipate:
- The uncertainty related to future science breakthroughs in the field
- The transformative effect anticipated breakthroughs may have on research and society
- The scope for action in the present in relation to anticipated breakthroughs.
This chart represents a summary of their responses to each of these elements, which when combined, provide the Anticipation Potential for the topic. See methodology for more information.
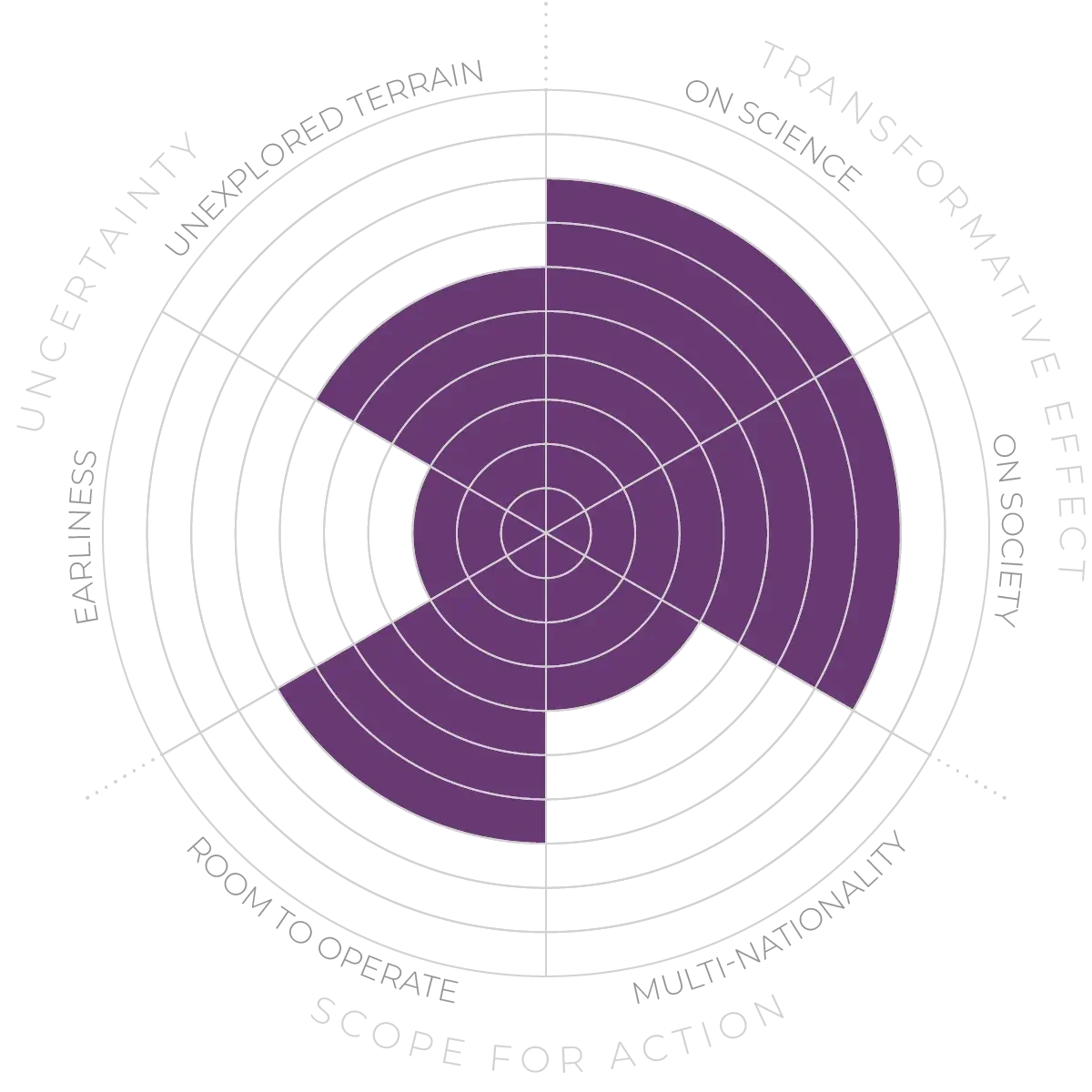