Future Horizons:
10-yearhorizon
AI accelerates performance and reliability of models across Earth Systems
25-yearhorizon
Model uncertainties are significantly reduced
An essential component of Earth-system science is therefore systematic comparison of “ensembles” of models.17 Efforts such as the Program for Climate Model Diagnosis and Intercomparison (PCMDI) co-ordinated at Lawrence Livermore National Laboratory have made headway in systematising the comparison process.18 This has enabled the use of the models in the Intergovernmental Panel on Climate Change (IPCC) assessment reports. In the most recent round of intercomparisons, CMIP6, researchers found that it was necessary to weight some models more strongly than others to give a more accurate ensemble than a simple average:19 optimising such weightings is a significant research problem.
An ongoing challenge for Earth-system modellers is to understand in which circumstances the Earth system is stable and resilient, and when it instead behaves chaotically or changes violently. Palaeoclimatologists have documented many sudden shifts in the climate: these include the 4.2ka BP event (a widespread east Mediterranean drought that may have lasted a century 4200 years ago) and the rapid temperature shifts known as Dansgaard-Oeschger events that punctuated the last glacial period. Consequently, some climatologists have suggested the models are unrealistically stable, although a lack of suitably configured models means that these conclusions are premature. It remains uncertain just how much natural instability the system possesses and how to represent this in models.
All these uncertainties would be reduced by improved gathering of observational data, better anchoring the models in reality. This seems a particularly urgent task because 2023 was exceptionally warm, to an extent that has so far eluded quantitative explanations.20
Model intercomparison - Anticipation Scores
The Anticipation Potential of a research field is determined by the capacity for impactful action in the present, considering possible future transformative breakthroughs in a field over a 25-year outlook. A field with a high Anticipation Potential, therefore, combines the potential range of future transformative possibilities engendered by a research area with a wide field of opportunities for action in the present. We asked researchers in the field to anticipate:
- The uncertainty related to future science breakthroughs in the field
- The transformative effect anticipated breakthroughs may have on research and society
- The scope for action in the present in relation to anticipated breakthroughs.
This chart represents a summary of their responses to each of these elements, which when combined, provide the Anticipation Potential for the topic. See methodology for more information.
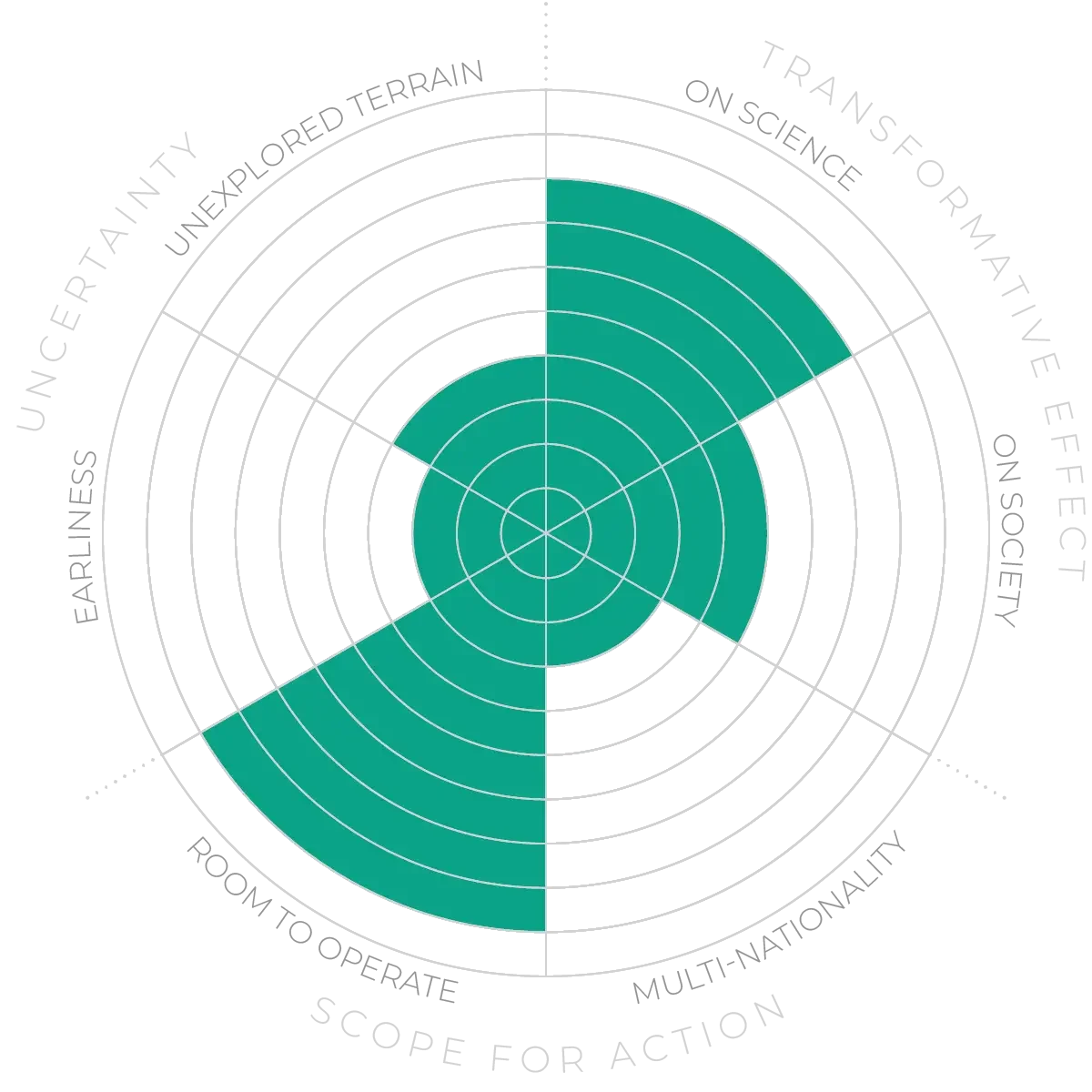