Future Horizons:
10-yearhorizon
The behavioural-science research environment matures
25-yearhorizon
Conflict prediction models adopted by global organisations
For example, analysis of 1.2 billion mobile-phone pings in South Africa reveals that contact with a larger number of immigrants predicts more xenophobic attitudes and voting patterns. However, this effect is less pronounced if there are repeated casual interactions with the same members of the out-group.2 Another “top-down” example is the use of artificial intelligence to categorise the way political leaders use language, thus revealing whether voters are hearing constructive debate or personal attacks.3
Data is not a panacea, however: there can be significant communities who are poorly represented in available data — for example, those who are not digitally connected — or hard to identify, such as those who are covertly resistant to autocratic regimes. And all the data in the world is of little use without the appropriate techniques for analysing it. These analytic approaches do not necessarily exist within the traditional social sciences and will need to be adapted and further developed in collaboration with specialists from other disciplines, such as physics and evolutionary biology.
Understanding intergroup dynamics - Anticipation Scores
The Anticipation Potential of a research field is determined by the capacity for impactful action in the present, considering possible future transformative breakthroughs in a field over a 25-year outlook. A field with a high Anticipation Potential, therefore, combines the potential range of future transformative possibilities engendered by a research area with a wide field of opportunities for action in the present. We asked researchers in the field to anticipate:
- The uncertainty related to future science breakthroughs in the field
- The transformative effect anticipated breakthroughs may have on research and society
- The scope for action in the present in relation to anticipated breakthroughs.
This chart represents a summary of their responses to each of these elements, which when combined, provide the Anticipation Potential for the topic. See methodology for more information.
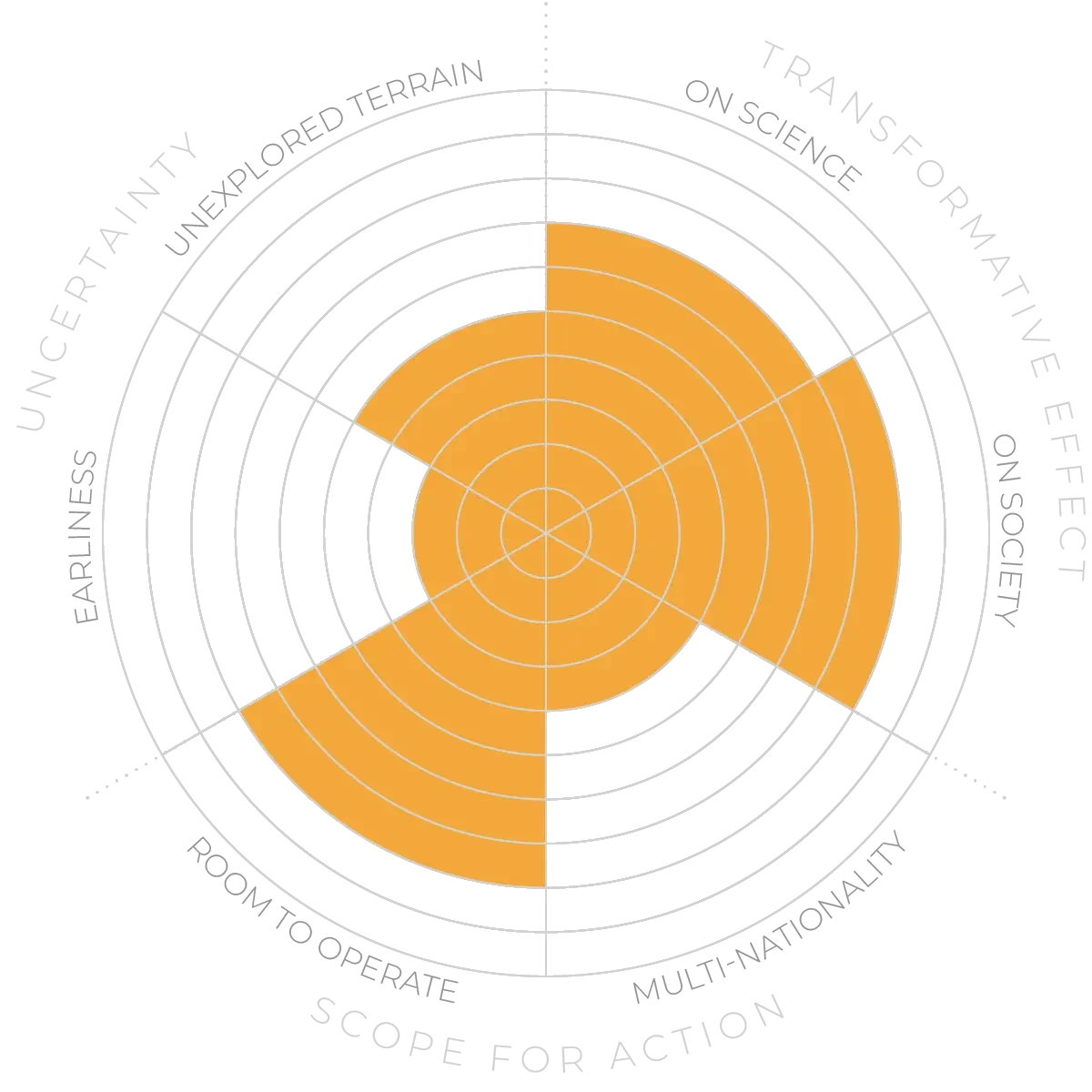