Future Horizons:
10-yearhorizon
Neuromorphic computing is developed for embodied AI
25-yearhorizon
Experiments demonstrate brain-like memory and logic
This requires the development of new kinds of computer chips that more faithfully mimic the way neurons work and are arranged.2 3 One particular challenge is to replicate how brain cells communicate via spikes of electrical activity, which is very different from how conventional processors operate. Current models of biological neurons and learning rules are also too simplistic, brushing over physiological details known to play an important role in the brain.
Nonetheless, large-scale neuromorphic systems are coming online. Intel’s new Hala Point system combines 1,152 of its Loihi 2 neuromorphic processors to emulate up to 1.15 billion neurons.4 And SpiNNcloud Systems is selling neuromorphic supercomputers based on chip designs pioneered by the SpiNNAker project at the University of Manchester, UK.5 6
There have also been breakthroughs in chips that carry out computation directly in memory.7 These are well-suited to implementing neuromorphic computing, but also holds promise for reducing deep-learning AI’s energy consumption. That could lead to growing convergence between the two fields.
However, biological realism is still limited. Breakthroughs in mapping neural connections in real brains and more biologically plausible learning approaches are helping close the gap.8 9 But faithfully replicating the brain’s function and structure in silicon remains a distant goal.
Neuromorphic Computing - Anticipation Scores
The Anticipation Potential of a research field is determined by the capacity for impactful action in the present, considering possible future transformative breakthroughs in a field over a 25-year outlook. A field with a high Anticipation Potential, therefore, combines the potential range of future transformative possibilities engendered by a research area with a wide field of opportunities for action in the present. We asked researchers in the field to anticipate:
- The uncertainty related to future science breakthroughs in the field
- The transformative effect anticipated breakthroughs may have on research and society
- The scope for action in the present in relation to anticipated breakthroughs.
This chart represents a summary of their responses to each of these elements, which when combined, provide the Anticipation Potential for the topic. See methodology for more information.
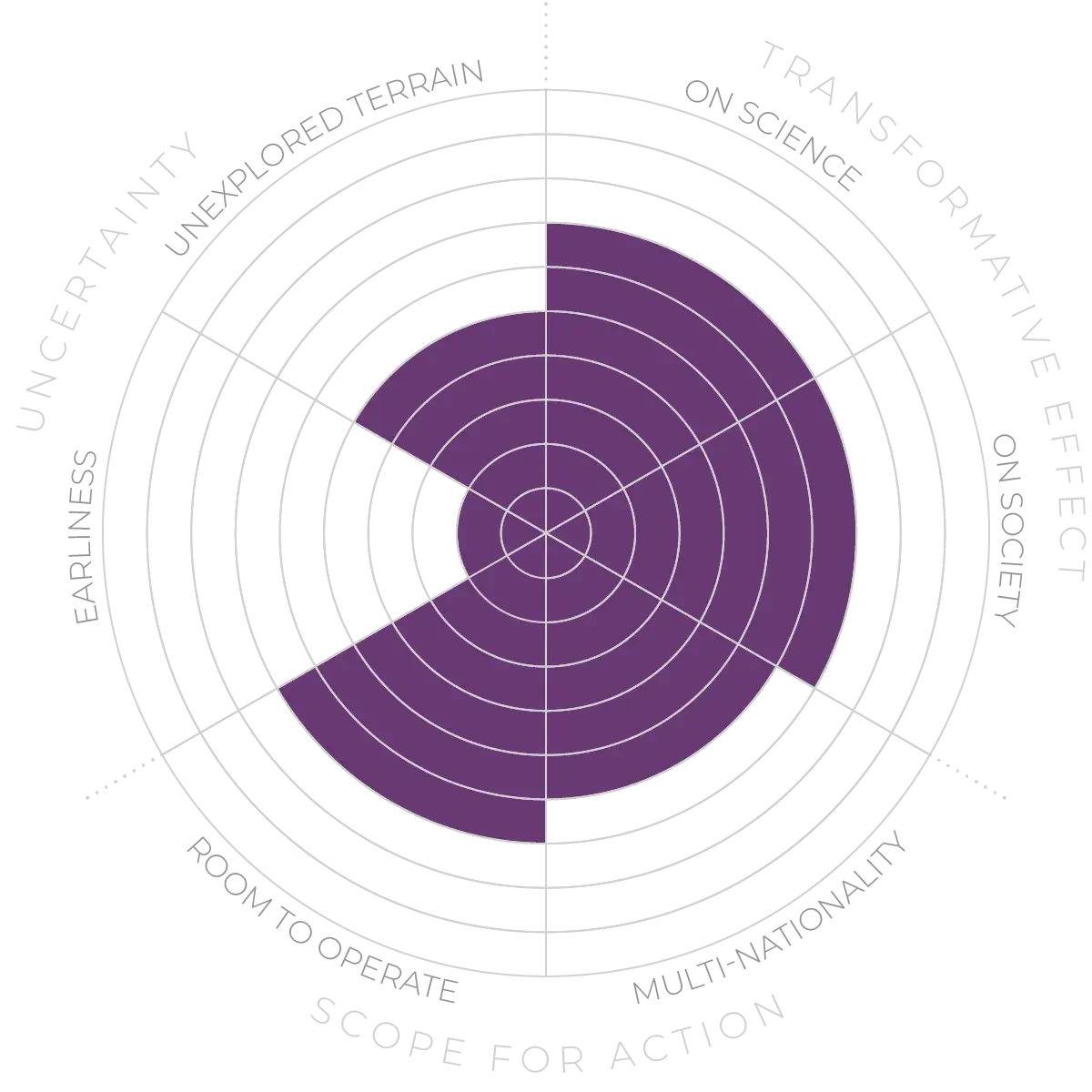