Future Horizons:
10-yearhorizon
AI deployments improve therapeutic success
25-yearhorizon
AI becomes a reliable drug-discovery partner
First, machine learning can identify the complicated "lock and key" relationships between new compounds and the relevant disease-related proteins they may affect. AI systems like AlphaFold, which can predict a protein's 3D structure from its sequence, are teaming up with major pharmaceutical companies to hasten the discovery of small-molecule therapeutics.32‘Alphabet’s Isomorphic stacks two new deals with Lilly’
Second, AI can mine the metagenome — the diversity of all existing natural molecules34‘Discovery of antimicrobial peptides in the global microbiome with machine learning’
Third, machine learning can be deployed to find patterns in other complex datasets, for example in the heaps of data generated during clinical trials, whether they succeed or fail.
But AI and machine learning are not magic tools, and are useless without good data. Data generation requires well-curated and standardised public data sets, and functionalising AI also requires next-generation manufacturing and regulatory approaches to bring synthesis into line with discovery.
Drug discovery - Anticipation Scores
The Anticipation Potential of a research field is determined by the capacity for impactful action in the present, considering possible future transformative breakthroughs in a field over a 25-year outlook. A field with a high Anticipation Potential, therefore, combines the potential range of future transformative possibilities engendered by a research area with a wide field of opportunities for action in the present. We asked researchers in the field to anticipate:
- The uncertainty related to future science breakthroughs in the field
- The transformative effect anticipated breakthroughs may have on research and society
- The scope for action in the present in relation to anticipated breakthroughs.
This chart represents a summary of their responses to each of these elements, which when combined, provide the Anticipation Potential for the topic. See methodology for more information.
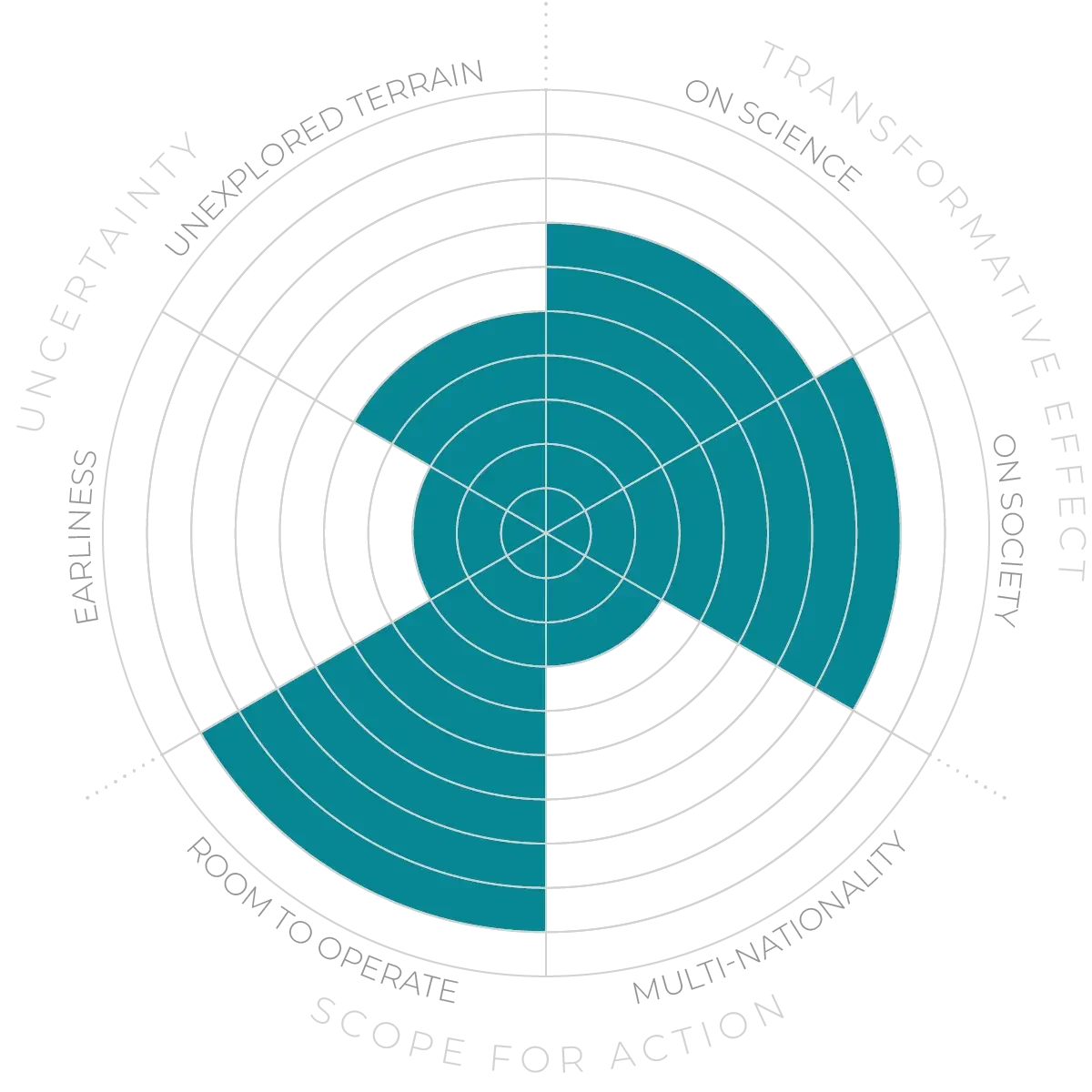